
Moreover, visual examination of LULC should be considered for choosing better classification approach as pixel-based approach produces salt-pepper effect in LULC, whereas OBC produces visually smoothened LULC and identifies even smaller objects in urban landscape. The results suggest that due to heterogeneity in urban land-use, it is difficult to achieve higher overall accuracy in classifying a large urban area using 5 m resolution satellite dataset. RF and OBC are identified as potential classifiers with majority of datasets. It has been observed that (1) the performance of all classification algorithms differs from each other and (2) resolution merged data impacts classification accuracy differently when compared to other satellite image of same spatial resolution. The accuracy assessment of the images is carried out using confusion matrix and Mc Nemar’s test. 27 LULC maps (9 classification algorithms * 3 images) are evaluated for comparing classification algorithms. High resolution (5 m) satellite image LISS 4 MX70, resolution merged satellite images (5 m) LISS 3 merged with LISS 4 mono and LISS 3 merged with IRS-1D are employed for classification. Additionally, the study has analysed the impact of different types of satellite datasets (i.e., high resolution image and resolution merged images of same resolution) on relative effectiveness of the algorithms in classifying the satellite imageries accurately. The algorithms include maximum likelihood, neural network, support vector machine (linear, polynomial, RBF (radial basis function), sigmoid kernels), random forest (RF) and Naive Bayes for pixel-based classification and maximum likelihood algorithm for object-based classification (OBC) approach. Hence, in the present study, nine different pixel- and object-based classification algorithms have been used to compare their relative effectiveness in generating remotely sensed LULC maps. However, still there is a lack of studies that can provide a comprehensive analysis on widely used classification algorithms. Literature offers studies comparing different classification algorithms/approaches to prepare LULC maps. The preparation of accurate LULC is of great importance as it is used in various studies ranging from change detection to geospatial modelling. It confirms that the main perspective of this problem is to combine different indicators from multiple sensors. This review shows that most of the state-of-the art research works focus on only one type of data. This paper overviews existing GNSS and on-board vision-based solutions of environmental context detection. Thus, it is important firstly to define this concept of context for navigation and to find a way to extract it from available information. That is why context detection is considered the future of navigation systems.
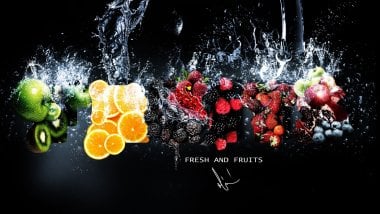
The context detection will enable us to build an adaptive navigation system to improve the precision and the robustness of its localization solution by anticipating possible degradation in sensor signal quality (GNSS in urban canyons for instance or camera-based navigation in a non-textured environment). For example, an object-based approach resulted in a 41.73% greater accuracy for the built area category, which is of particular importance to WUI wildfire mitigation.Ĭurrent navigation systems use multi-sensor data to improve the localization accuracy, but often without certitude on the quality of those measurements in certain situations. Results indicate that an object-based classification approach provides a higher accuracy than a pixel-based classification approach when distinguishing between the selected land-use and land-cover categories. Here we explore the accuracy of pixel-based and object-based classification methods used for mapping in the wildland–urban interface (WUI) with free, readily available, high spatial resolution urban imagery, which is available in many places to municipal and local fire management agencies.
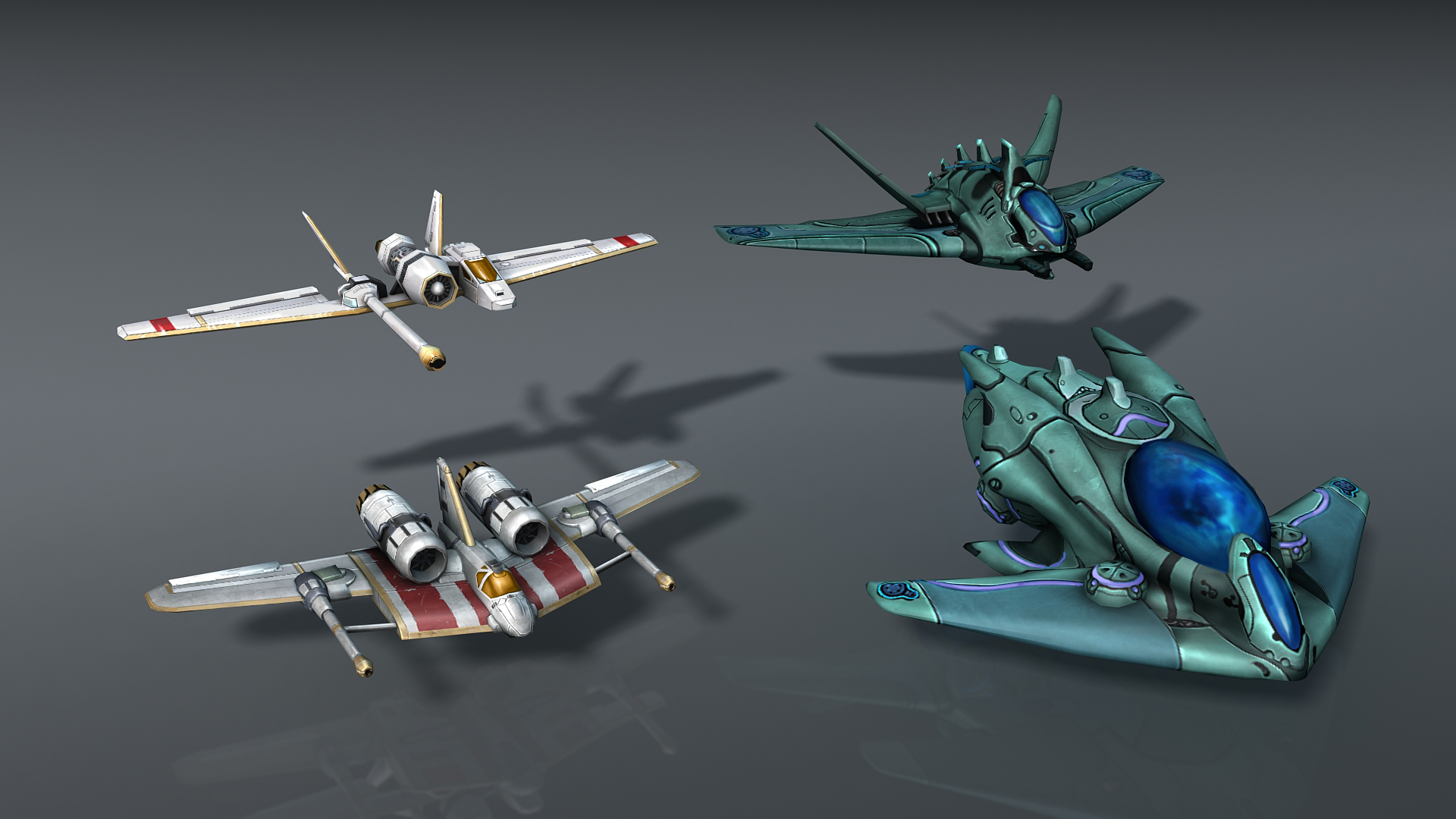
However, some image processing approaches may be more appropriate than others in distinguishing land-use and land-cover categories, particularly when classifying high spatial resolution imagery for urbanizing environments. Land-use and land-cover maps can assist decision-makers in targeting and prioritizing risk mitigation activities, and remote sensing techniques provide effective and efficient methods to create such maps. The expansion of urban development into wildland areas can have significant consequences, including an increase in the risk of structural damage from wildfire.
